Don’t Get Fooled By Data
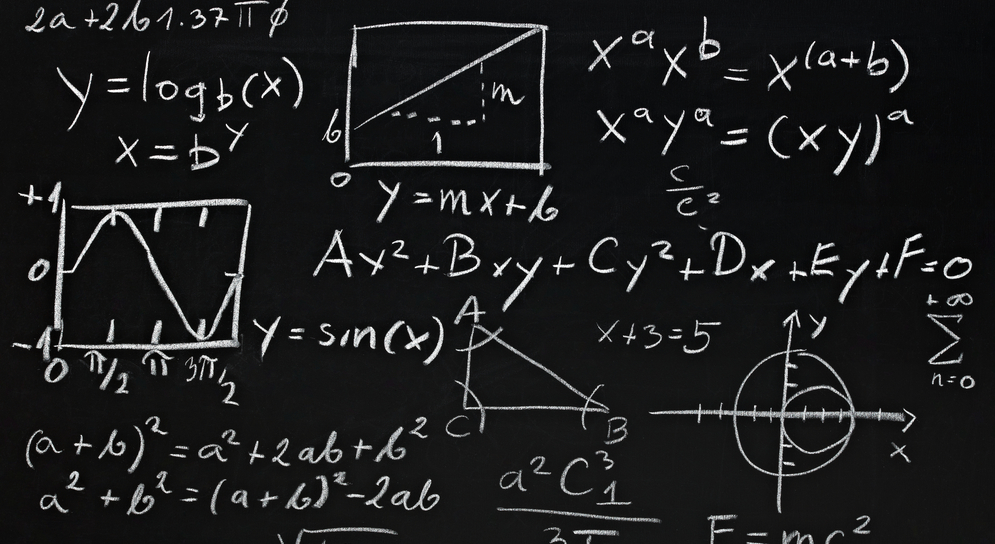
April Fool’s is around the corner…and nectarOM has a few suggestions to reduce your risk of getting fooled by data analysis.
In a time when data is abundant and necessary for a strong personalized marketing strategy, marketers should be on the look out for these most common ways that data is misinterpreted. The following are some mistakes commonly made in data analysis.
Causation and Correlation
Understanding the difference between causation and correlation is important to interpreting data. Because both concepts sound relatively similar and are related to statistics, they are easily confused for one another.
Causation occurs when one event causes another. For example, as summer approaches, a swimwear retailer may see an increase in sales as more people buy swimsuits.
Correlation occurs when there is a mutual relation between two events. However, one of these events does not necessarily need to cause the other. For example, ice cream sales may increase and a swimwear retailer’s sales may increase, however, this does not mean that the increase in ice cream sales causes people to buy more swimwear. In this case, the rise in temperature is the cause of both of these events.
Understanding the difference between causation and correlation is important to avoid an incorrect data analysis. If the aforementioned swimwear retailer confuses its correlation and causation with ice cream sales, the retailer may see problems arise if it adjusts its marketing campaign to reflect the success of ice cream sales. For example, if summer ice cream sales increase because a neighboring frozen yogurt shop shuts down, the swimwear retailer may wrongfully assume their sales will increase as well. This assumption, which is not necessarily correct, could contribute to an ineffective marketing campaign.
Using Old Data
When a company is stuck with outdated customer information, its data may become useless. For example, a company may be sending emails to a customer’s old email address. If this customer no longer checks this email address, he or she will not have the opportunity to open emails from companies they might have registered with. This could alter the company’s email open rates. Several cases of this could lead to incorrect assumptions of ineffective subject lines or poor sending times based off faulty data collected in the scenario. To prevent these types of inaccurate assumptions, analysts should ensure they are using current customer information and use business rules to exclude customers who have not opened within a certain period of time.
Assuming the Data Will Do it All
One of the attractive selling points of using a Data Management Platform is that it reduces work for marketers and analysts. However, this mindset is a slippery slope. Companies should make sure their staff knows that a DMP doesn’t mean no more work in personalizing and customer care. Marketers can simply sit back and let their DMP run their data analysis and marketing campaigns. Marketers must remain attentive and responsive to consumer behavior, ensuring that marketing does not take on a robotic, impersonal feel.
Measuring the Average
When determining metrics in a data set, marketers must determine how to measure an accurate average. In some data sets, using mean versus median can present some vastly different results.
Mean accounts a total of all values added, then divided by the amount of data points. Median is the exact middle of the data set in numerical order. In cases where there are extreme outliers, using the median can give analysts a better picture of an average.
Oftentimes, the median gives marketers a more accurate look at its average. For example, consider a retailer’s data that tracks how long visitors stay on their eCommerce site. Imagine a retailer’s data shows that nine users spend 3 minutes on its site, while one user spends 45 minutes on the site. In this scenario, the mean average is 7.2 minutes spent on site, while the median is 3 minutes on site.
The median is a better value for the retailer’s average because it shows reflects a value that is close to what most site visitors showed. In contrast, the mean average reflects a value significantly higher than what 9 out of the 10 visitors generated. The mean’s higher value is skewed by one user’s unusually high value of 45 minutes. This lone value seriously alters the average time spent on the site.
Acknowledging outside factors
Oftentimes, marketers are so focused on the numbers that they forget to account for outside factors that might influence their customer data. For example, when looking at open rates in an automated email campaign, marketers should be sure to consider a customer’s geographical location.
While geographical location might seem irrelevant when sending emails, time zones and time of distribution can significantly impact open rates. Studies show that most consumers open emails from retailers between 2 p.m. and 5 p.m.
If all emails are distributed at the same time, a person in California might receive the message at an optimal time of 4 p.m., while a recipient in New York would receive the same exact message at their time of 7 p.m. While this delivery time is great for the Californian, the New Yorker may be in the middle of dinner and too distracted to open an email. Content should be delivered with the recipient’s location – and time zone – in mind.
Consider Kate Spade’s automated email campaign, which always considers the shopper’s time zone when delivering emails. The women’s clothing brand asks its registrants for two items of information upon signing up for an account: their email and zip code. With this information, Kate Spade emails customers according to their different time zones.
The email to the left is registered with under my California zip code, while the email to the right was registered under my Texas zip code. I received both emails two hours apart – a perfect example of a company accounting for time zone differences.
While data analysis mistakes are bad for marketers, poor data management can be detrimental to a company’s growth and sales as well. Make sure your company’s data analysis and data management are up-to-date and set up for success when implementing data into your marketing campaign.